New AI model is a leap for autonomous materials science
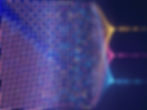
Materials science enables cutting-edge technologies, from lightweight cars and powerful computers to high-capacity batteries and durable spacecraft. But to develop materials for these applications, they need to be exactingly analyzed through numerous microscopic lenses—a difficult and time-consuming process.
A new artificial intelligence (AI) model developed at Pacific Northwest National Laboratory (PNNL) can identify patterns in electron microscope images of materials without requiring human intervention, allowing for more accurate and consistent materials science. It also removes a barrier for autonomous experimentation on electron microscopes—an important component of so-called “self-driving labs.”
“We do a lot of different materials science at the lab, whether developing new materials for catalysts, energy storage, or electronics,” said Steven Spurgeon, a senior materials scientist at PNNL who has been working to apply AI in materials science for many years. “We also do a lot of work in understanding how materials evolve in different environments. If you put—for example—sensors in a nuclear reactor or a spacecraft, they’re going to be exposed to high-radiation environments, leading to degradation over time.”
Understanding that degradation, in turn, helps researchers design better materials.
Typically, to train an AI model to understand a phenomenon like radiation damage, researchers would painstakingly produce a hand-labeled training dataset, manually tracing the radiation-damaged regions on electron microscope images. That hand-labeled dataset would then be used to train an AI model, which would identify the shared characteristics of those human-identified regions and seek to identify similar regions in unlabeled images.
Labeling datasets by hand is not ideal. It is a time-consuming process—but moreover, humans are more prone to inconsistencies and inaccuracies in their labeling, and they’re not as good at simultaneously considering (and even-handedly labeling) different lenses (modalities) of the same sample. “Typically, the human is making subjective assessments of the data,” Spurgeon said. “And we just can’t do that with the types of hardware we’re building now.”
Using labeled data also requires a human “in the loop,” pausing the experimentation process as humans interpret or label the data from a new electron microscope image.
The solution: an unsupervised model that is able to analyze the data without involving humans.
Taking off the training wheels
“What we wanted to do is to come up with an unsupervised approach to classifying electron microscope imagery,” said Arman Ter-Petrosyan, a research associate at PNNL. “And beyond the basic problem of classification, we wanted to come up with ways to use these models to describe different material interfaces.”
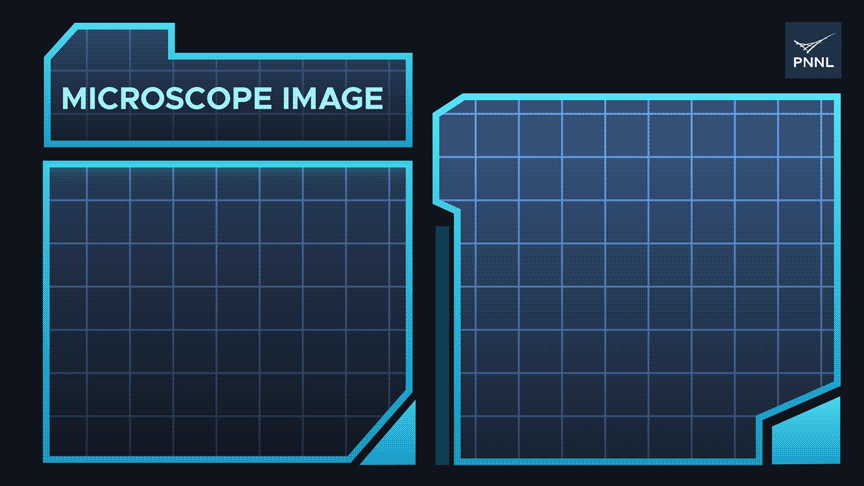
The team began with the ResNet50 AI model and a preexisting dataset of over 100,000 unlabeled electron microscopy images called MicroNet. Using that as a foundation, they taught the model to divide each electron microscope image into a grid of small “chips,” then instructed it to calculate the overall similarities between chips and assign them similarity scores to one another. Groups of chips that are most similar to one another are then sorted into “communities” that represent parts of the image with comparable features.
The result is an abstract representation of patterns in the data that can then be dispersed back across the electron microscope images and color-coding regions by their respective communities—all without requiring a human to tell the model what to look for.
The researchers have been applying the new model to understand radiation damage in materials that are used in high-radiation environments like nuclear reactors. The model is able to accurately “chip” the degraded areas and sort the image into communities representing different levels of radiation damage.
“This is a way of taking the data and representing relationships among areas that aren’t necessarily next to each other in the material,” Ter-Petrosyan explained.
Better than human
The beauty of the model, the researchers explained, is that it identifies these communities with extraordinary consistency, producing the outlined regions of labeled data without any of the mercurial deviations of human labeling. This is helpful not just for assessing an image but also for establishing objective metrics to describe different states of materials.
“I have a perfect material; I irradiate it; it starts to break down,” Spurgeon said. “How do I describe that process so that I can engineer that material better for a particular application? Our problem is that we have the data—we’ve had it for a long time!—and we’re able to collect it routinely, but we’re not using it to get those descriptors out.”
What is more, electron microscopes capture more than just one image at a time—actually, they capture various images, spectroscopy readings, and diffraction patterns. But with human labeling, datasets and AI models are almost always limited to identifying patterns across just one type of data (or “modality”).
But now, with unsupervised AI, the door is open for multimodal models that simultaneously incorporate multiple lenses of data. “The more types of data you add, the more powerful and more predictive your model becomes,” Spurgeon said.
Autonomous experimentation
This development is another step toward robust, autonomous material experimentation on electron microscopes at PNNL. The Lab’s innovative AutoEM (Artificial Intelligence-Guided Transmission Electron Microscope) project had already been able to use AI to merge and identify features in electron microscope imagery on the fly, allowing researchers to select points of interest that are then intelligently investigated by AutoEM.
AutoEM is a unique microscope platform that combines the power of machine learning with advanced automation to probe the building blocks of matter with unprecedented speed, clarity, and precision. @ Pacific Northwest National Laboratory
The new model expands those capabilities, enabling the rapid detection and categorization of similar regions and trends. “A lot of this is already deployed on multiple microscopes at PNNL,” Spurgeon said.
Now, the researchers will work on tuning the model to understand new modalities of data as well as different and more complex phenomena. They are also working on speeding up the model so that it can be used in real time as the electron microscopes produce data.
“Moving forward, we really want to demonstrate how this can be done practically,” Spurgeon said. “It’s not just a model we’re running offline—it’s being used by people at the time of our experiments. Hopefully, that establishes a prototype for other people in the community.”
Reference Unsupervised segmentation of irradiation‐induced order‐disorder phase transitions in electron microscopy
Arman H Ter-Petrosyan, Jenna A Bilbrey, Christina M Doty, Bethany E Matthews, Le Wang, Yingge Du, Eric Lang, Khalid Hattar, Steven R Spurgeon